#publication
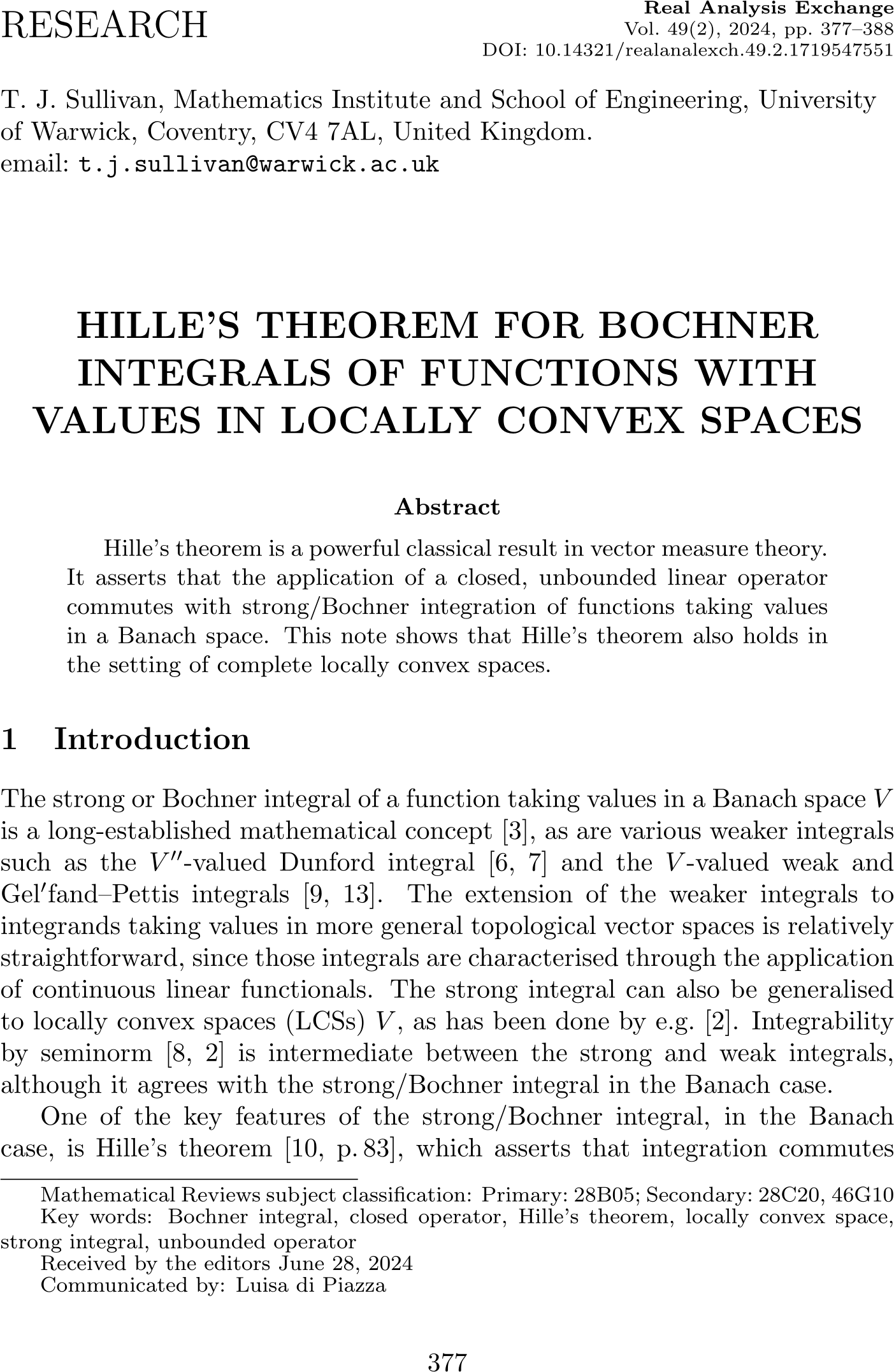
Hille's theorem for locally convex spaces in Real Analysis Exchange
The article “Hille's theorem for Bochner integrals of functions with values in locally convex spaces” has just appeared in its final form in Real Analysis Exchange.
T. J. Sullivan. “Hille's theorem for Bochner integrals of functions with values in locally convex spaces.” Real Analysis Exchange 49(2):377–388, 2024.
Abstract. Hille's theorem is a powerful classical result in vector measure theory. It asserts that the application of a closed, unbounded linear operator commutes with strong/Bochner integration of functions taking values in a Banach space. This note shows that Hille's theorem also holds in the setting of complete locally convex spaces.
Published on Tuesday 1 October 2024 at 13:00 UTC #publication #real-anal-exch #functional-analysis #hille-theorem
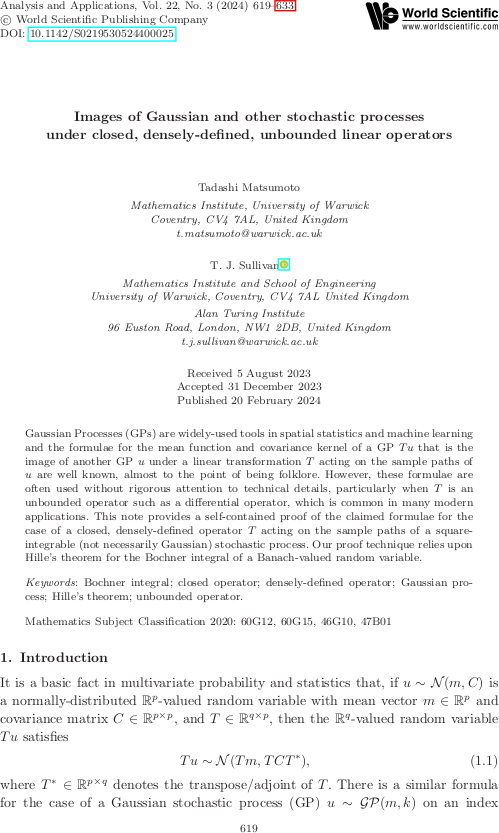
Unbounded images of Gaussian and other stochastic processes in Analysis and Applications
The final version of “Images of Gaussian and other stochastic processes under closed, densely-defined, unbounded linear operators” by Tadashi Matsumoto and myself has just appeared in Analysis and Applications.
The purpose of this article is to provide a self-contained rigorous proof of the well-known formula for the mean and covariance function of a stochastic process — in particular, a Gaussian process — when it is acted upon by an unbounded linear operator such as an ordinary or partial differential operator, as used in probabilistic approaches to the solution of ODEs and PDEs. This result is easy to establish in the case of a bounded operator, but the unbounded case requires a careful application of Hille's theorem for the Bochner integral of a Banach-valued random variable.
T. Matsumoto and T. J. Sullivan. “Images of Gaussian and other stochastic processes under closed, densely-defined, unbounded linear operators.” Analysis and Applications 22(3):619–633, 2024.
Abstract. Gaussian processes (GPs) are widely-used tools in spatial statistics and machine learning and the formulae for the mean function and covariance kernel of a GP \(v\) that is the image of another GP \(u\) under a linear transformation \(T\) acting on the sample paths of \(u\) are well known, almost to the point of being folklore. However, these formulae are often used without rigorous attention to technical details, particularly when \(T\) is an unbounded operator such as a differential operator, which is common in several modern applications. This note provides a self-contained proof of the claimed formulae for the case of a closed, densely-defined operator \(T\) acting on the sample paths of a square-integrable stochastic process. Our proof technique relies upon Hille's theorem for the Bochner integral of a Banach-valued random variable.
Published on Wednesday 21 February 2024 at 10:00 UTC #publication #anal-appl #prob-num #gp #matsumoto
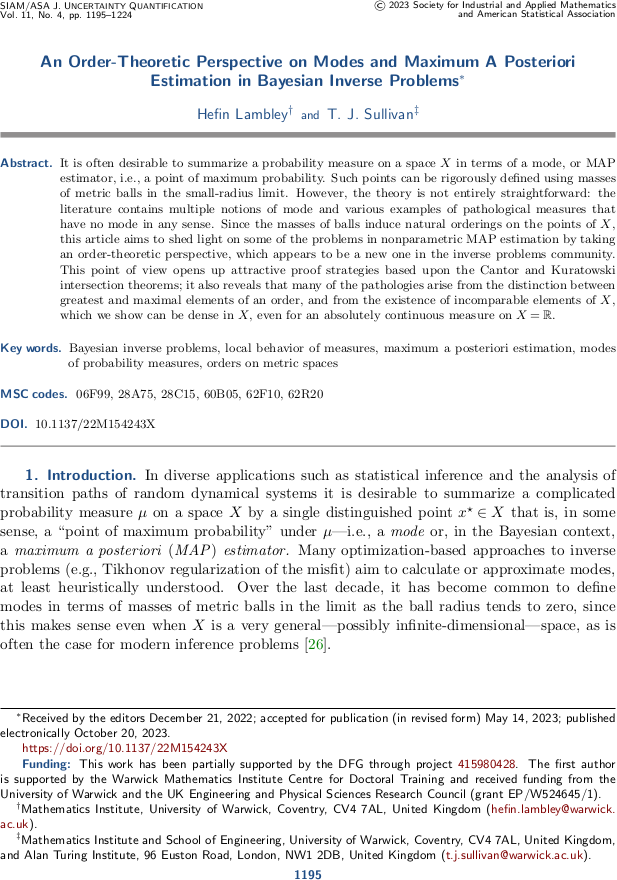
Order-theoretic perspectives on MAP estimation in SIAM/ASA JUQ
The final version of “An order-theoretic perspective on modes and maximum a posteriori estimation in Bayesian inverse problems” by Hefin Lambley and myself has just appeared online in the SIAM/ASA Journal on Uncertainty Quantification.
On a heuristic level, modes and MAP estimators are intended to be the “most probable points” of a space \(X\) with respect to a probability measure \(\mu\). Thus, in some sense, they would seem to be the greatest elements of some order on \(X\), and a rigorous order-theoretic treatment is called for, especially for cases in which \(X\) is, say, an infinite-dimensional function space. Such an order-theoretic perspective opens up some attractive proof strategies for the existence of modes and MAP estimators but also leads to some interesting counterexamples. In particular, because the orders involved are not total, some pairs of points of \(X\) can be incomparable (i.e. neither is more nor less likely than the other). In fact we show that there are examples for which the collection of such mutually incomparable elements is dense in \(X\).
H. Lambley and T. J. Sullivan. “An order-theoretic perspective on modes and maximum a posteriori estimation in Bayesian inverse problems.” SIAM/ASA Journal on Uncertainty Quantification 11(4):1195–1224, 2023.
Published on Friday 20 October 2023 at 09:00 UTC #publication #modes #order-theory #map-estimators #lambley #juq
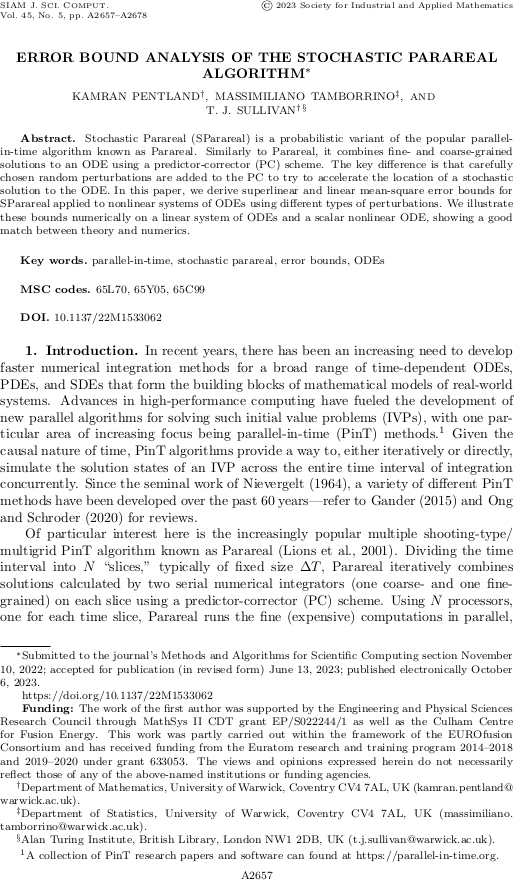
Error analysis for SParareal in SISC
The final version of “Error bound analysis of the stochastic parareal algorithm” by Kamran Pentland, Massimiliano Tamborrino, and myself has just appeared online in the SIAM Journal on Scientific Computing (SISC).
K. Pentland, M. Tamborrino, and T. J. Sullivan. “Error bound analysis of the stochastic parareal algorithm.” SIAM Journal on Scientific Computing 45(5):A2657–A2678, 2023.
Abstract. Stochastic Parareal (SParareal) is a probabilistic variant of the popular parallel-in-time algorithm known as Parareal. Similarly to Parareal, it combines fine- and coarse-grained solutions to an ODE using a predictor-corrector (PC) scheme. The key difference is that carefully chosen random perturbations are added to the PC to try to accelerate the location of a stochastic solution to the ODE. In this paper, we derive superlinear and linear mean-square error bounds for SParareal applied to nonlinear systems of ODEs using different types of perturbations. We illustrate these bounds numerically on a linear system of ODEs and a scalar nonlinear ODE, showing a good match between theory and numerics.
Published on Monday 9 October 2023 at 09:00 UTC #publication #prob-num #sparareal #pentland #tamborrino #sisc
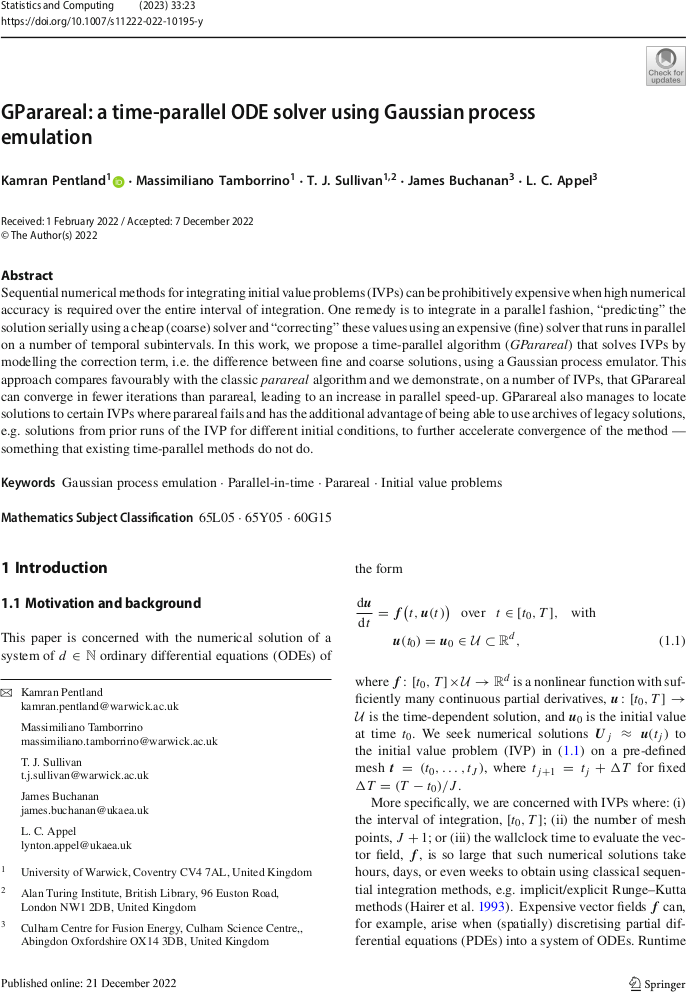
GParareal in Statistics and Computing
The article “GParareal: A time-parallel ODE solver using Gaussian process emulation” by Kamran Pentland, Massimiliano Tamborrino, James Buchanan, Lynton Appel and myself has just been published in its final form in Statistics and Computing. In this paper, we show how a Gaussian process emulator for the difference between coarse/cheap and fine/expensive solvers for a dynamical system can be used to enable rapid and accurate solution of that dynamical system in a way that is parallel in time. This approach extends the now-classical Parareal algorithm in a probabilistic way that allows for efficient use of both runtime and legacy data gathered about the coarse and fine solvers, which may be a critical performance advantage for complex dynamical systems for which the fine solver is too expensive to run in series over the full time domain.
K. Pentland, M. Tamborrino, T. J. Sullivan, J. Buchanan, and L. C. Appel. “GParareal: A time-parallel ODE solver using Gaussian process emulation.” Statistics and Computing 33(1):no. 20, 23pp., 2023.
Abstract. Sequential numerical methods for integrating initial value problems (IVPs) can be prohibitively expensive when high numerical accuracy is required over the entire interval of integration. One remedy is to integrate in a parallel fashion, “predicting” the solution serially using a cheap (coarse) solver and “correcting” these values using an expensive (fine) solver that runs in parallel on a number of temporal subintervals. In this work, we propose a time-parallel algorithm (GParareal) that solves IVPs by modelling the correction term, i.e. the difference between fine and coarse solutions, using a Gaussian process emulator. This approach compares favourably with the classic parareal algorithm and we demonstrate, on a number of IVPs, that GParareal can converge in fewer iterations than parareal, leading to an increase in parallel speed-up. GParareal also manages to locate solutions to certain IVPs where parareal fails and has the additional advantage of being able to use archives of legacy solutions, e.g. solutions from prior runs of the IVP for different initial conditions, to further accelerate convergence of the method - something that existing time-parallel methods do not do.
Published on Thursday 22 December 2022 at 12:00 UTC #publication #prob-num #pentland #tamborrino #buchanan #appel