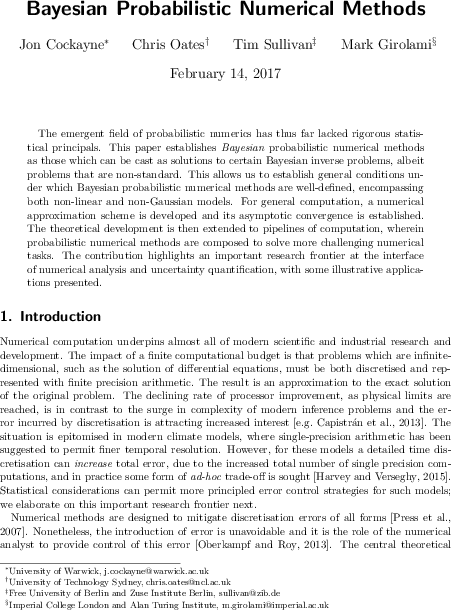
Bayesian probabilistic numerical methods
Jon Cockayne, Chris Oates, Mark Girolami and I have just uploaded a preprint of our latest paper, “Bayesian probabilistic numerical methods” to the arXiv. Following on from our earlier work “Probabilistic meshless methods for partial differential equations and Bayesian inverse problems”, our aim is to provide some rigorous theoretical underpinnings for the emerging field of probabilistic numerics, and in particular to define what it means for such a method to be “Bayesian”, by connecting with the established theories of Bayesian inversion and disintegration of measures.
Abstract. The emergent field of probabilistic numerics has thus far lacked rigorous statistical principals. This paper establishes Bayesian probabilistic numerical methods as those which can be cast as solutions to certain Bayesian inverse problems, albeit problems that are non-standard. This allows us to establish general conditions under which Bayesian probabilistic numerical methods are well-defined, encompassing both non-linear and non-Gaussian models. For general computation, a numerical approximation scheme is developed and its asymptotic convergence is established. The theoretical development is then extended to pipelines of computation, wherein probabilistic numerical methods are composed to solve more challenging numerical tasks. The contribution highlights an important research frontier at the interface of numerical analysis and uncertainty quantification, with some illustrative applications presented.
Published on Tuesday 14 February 2017 at 12:00 UTC #preprint #prob-num #bayesian #cockayne #oates #girolami