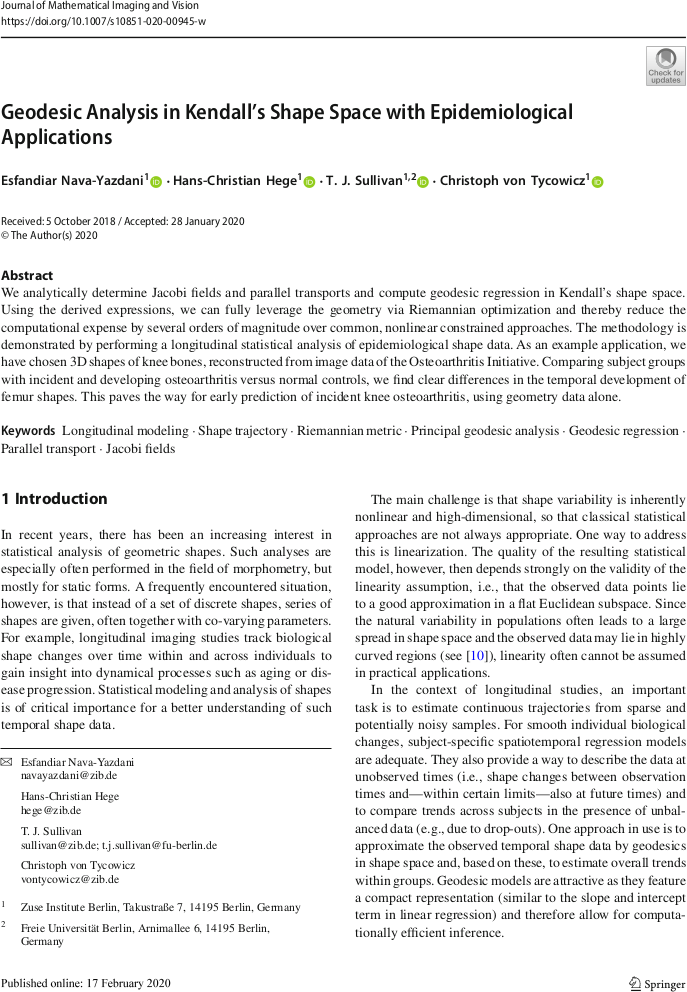
Geodesic analysis in Kendall's shape space now in J. Math. Imaging Vis.
The article “Geodesic analysis in Kendall's shape space with epidemiological applications” by Esfandiar Nava-Yazdani, Christoph von Tycowicz, Christian Hege, and myself has just appeared online in the Journal of Mathematical Imaging and Vision.
E. Nava-Yazdani, H.-C. Hege, T. J. Sullivan, and C. von Tycowicz. “Geodesic analysis in Kendall's shape space with epidemiological applications.” Journal of Mathematical Imaging and Vision 62(4):549–559, 2020.
Abstract. We analytically determine Jacobi fields and parallel transports and compute geodesic regression in Kendall’s shape space. Using the derived expressions, we can fully leverage the geometry via Riemannian optimization and thereby reduce the computational expense by several orders of magnitude over common, nonlinear constrained approaches. The methodology is demonstrated by performing a longitudinal statistical analysis of epidemiological shape data. As an example application, we have chosen 3D shapes of knee bones, reconstructed from image data of the Osteoarthritis Initiative. Comparing subject groups with incident and developing osteoarthritis versus normal controls, we find clear differences in the temporal development of femur shapes. This paves the way for early prediction of incident knee osteoarthritis, using geometry data alone.
Published on Tuesday 18 February 2020 at 14:00 UTC #publication #ch15 #shape-trajectories #nava-yazdani #von-tycowicz #hege