#mcmc
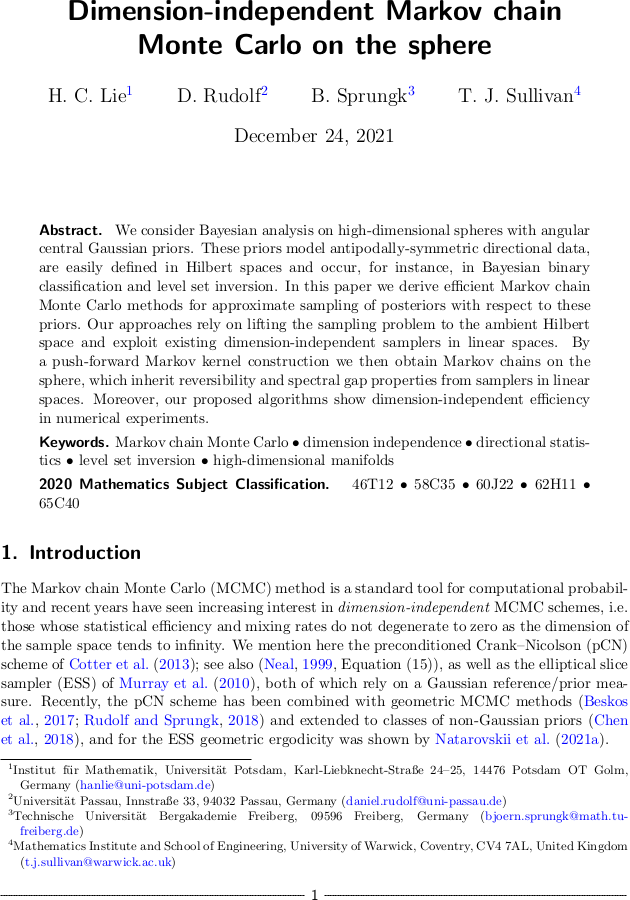
Dimension-independent MCMC on spheres
Han Cheng Lie, Daniel Rudolf, Björn Sprungk and I have just uploaded a preprint of our latest article, “Dimension-independent Markov chain Monte Carlo on the sphere”, to the arXiv. In this paper, motivated by problems such as Bayesian binary classification over continuous spaces, for which the parameter space is naturally an infinite-dimensional sphere of functions, we consider MCMC methods for inference on spheres of Hilbert spaces. In particular, we construct MCMC methods that have dimension-independent performance in terms of their acceptance probability, spectral gap, etc.; we also show how more naive approaches may lack basic properties such as Markovianity and reversibility; how how even sophisticated geometric MCMC approaches can still suffer from the curse of dimension.
Abstract. We consider Bayesian analysis on high-dimensional spheres with angular central Gaussian priors. These priors model antipodally-symmetric directional data, are easily defined in Hilbert spaces and occur, for instance, in Bayesian binary classification and level set inversion. In this paper we derive efficient Markov chain Monte Carlo methods for approximate sampling of posteriors with respect to these priors. Our approaches rely on lifting the sampling problem to the ambient Hilbert space and exploit existing dimension-independent samplers in linear spaces. By a push-forward Markov kernel construction we then obtain Markov chains on the sphere, which inherit reversibility and spectral gap properties from samplers in linear spaces. Moreover, our proposed algorithms show dimension-independent efficiency in numerical experiments.
Published on Wednesday 22 December 2021 at 12:00 UTC #preprint #mcmc #lie #rudolf #sprungk
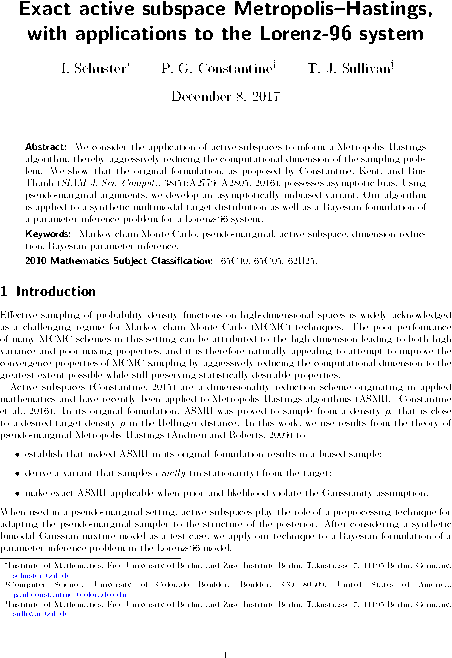
Active subspace Metropolis-Hastings
Ingmar Schuster, Paul Constantine, and I have just uploaded a preprint of our latest article, “Exact active subspace Metropolis–Hastings, with applications to the Lorenz-96 system”, to the arXiv. This paper reports on our first investigations into the acceleration of Markov chain Monte Carlo methods using active subspaces as compared to other adaptivity techniques, and is supported by the DFG through SFB 1114 Scaling Cascades in Complex Systems.
Abstract. We consider the application of active subspaces to inform a Metropolis–Hastings algorithm, thereby aggressively reducing the computational dimension of the sampling problem. We show that the original formulation, as proposed by Constantine, Kent, and Bui-Thanh (SIAM J. Sci. Comput., 38(5):A2779–A2805, 2016), possesses asymptotic bias. Using pseudo-marginal arguments, we develop an asymptotically unbiased variant. Our algorithm is applied to a synthetic multimodal target distribution as well as a Bayesian formulation of a parameter inference problem for a Lorenz-96 system.
Published on Friday 8 December 2017 at 08:00 UTC #preprint #mcmc #sfb1114 #schuster #constantine