#sfb1294
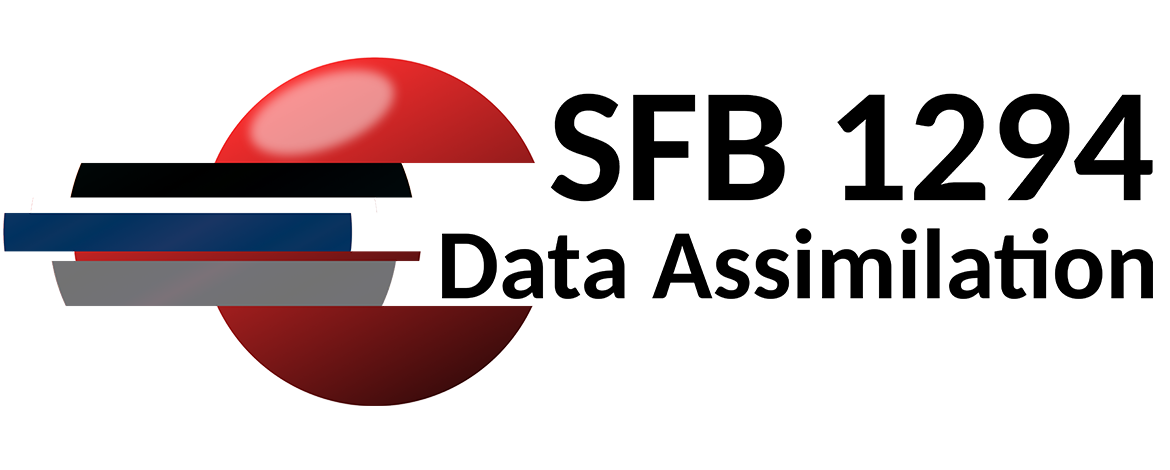
Kalman Lecture by Andrew Stuart at SFB1294
Andrew Stuart (Caltech) will give the inaugural Kalman Lecture of SFB 1294 Data Assimilation on the topic of “Large Graph Limits of Learning Algorithms”.
Time and Place. Friday 24 August 2018, 10:15–11:45, University of Potsdam, Campus Golm, Building 27, Lecture Hall 0.01
Abstract. Many problems in machine learning require the classification of high dimensional data. One methodology to approach such problems is to construct a graph whose vertices are identified with data points, with edges weighted according to some measure of affinity between the data points. Algorithms such as spectral clustering, probit classification and the Bayesian level set method can all be applied in this setting. The goal of the talk is to describe these algorithms for classification, and analyze them in the limit of large data sets. Doing so leads to interesting problems in the calculus of variations, in stochastic partial differential equations and in Monte Carlo Markov Chain, all of which will be highlighted in the talk. These limiting problems give insight into the structure of the classification problem, and algorithms for it.
Published on Friday 3 August 2018 at 11:00 UTC #event #sfb1294 #stuart
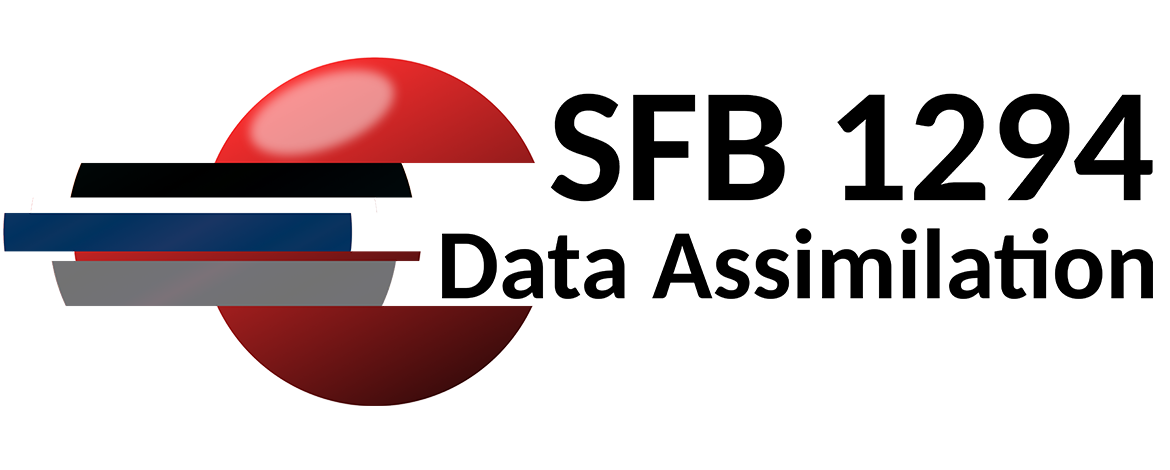
SFB1294 Colloquium
Next week it will be a great pleasure to give the SFB 1294 Data Assimilation colloquium talk on the topic of “Distributional Uncertainty in Uncertainty Quantification”.
Time and Place. Friday 8 December 2017, 10:15–11:45, University of Potsdam, Campus Golm, Building 28, Lecture Hall 108
Abstract. Many problems in forward and inverse uncertainty quantification assume a single probability distribution of interest, e.g. a distribution of random inputs or a prior measure for Bayesian inference. However, on close inspection, many of these probability distributions are not completely determined by the available information, and this introduces an additional source of uncertainty. For example there may be good grounds for assuming a particular form for the distribution, but the "correct" values of a few parameters may be known only approximately; at another extreme, only a few moments or statistics of the distribution may be known, leaving an infinite-dimensional non-parametric distributional uncertainty to be reckoned with.
Such so-called distributional or Knightian uncertainties may be particularly important if critical features of the system depend upon underdetermined aspects of the probability distribution such as tail behaviour. This talk will give a brief introduction to the treatment of such uncertainties, in both finite- and infinite-dimensional settings, including maximum entropy and optimisation approaches.
Published on Friday 1 December 2017 at 08:00 UTC #event #sfb1294